Design needs AI and AI needs us
- Simbiat
- Oct 21, 2023
- 7 min read
Updated: Nov 11, 2024
What is the relationship like between Design and Artificial Intelligence within your organisation? Are they parents that don't talk, separated by complex data algorithms and machine learning jargon? Are they distant relatives, reuniting briefly at the bottom of the service blueprint every so often? Or is there no relationship at all? Are they simply two ships passing late in the night? This article explores not only how AI can help us design better services, but also how design can help build better AI. The relationship is symbiotic.

How can AI help us design better services?
Artificial intelligence and machine learning are not only driving a new paradigm of data-driven, personalised experiences for users, but they are also creating new possibilities for designers too.
Lower barrier to research
Humanity is faced with a 'data rate problem'. We physically cannot process information as fast as machines, which means that whilst the benefits of research are clear, the time and money required make it increasingly difficult to get business buy-in. At times, this barrier leads to user research being omitted from the design process completely, resulting in frustrating or poorly-designed experiences. However, with natural language processing, hundreds of user interviews can be transcribed and analysed in real-time. Lengthy handwritten user diary studies can be deciphered easily in any language with advanced image detection. Numerous, overlapping insights that arise out of large focus groups can be aggregated quickly at an individual profile level using voice recognition, sentiment analysis and keyword extraction. This is not about AI replacing design jobs, but rather AI making the collation and interpretation of data easier for us. By augmenting our abilities, designers can identify opportunities sooner and understand user needs much earlier in the process.
Unbiased personas
There is a lot of talk about AI bias, but what most of us fail to realise is that responsible AI models can actually help us to address our own existing human biases. The UK design industry is 79 percent male and 86 percent white. A shortage of diverse perspectives and lived experiences unconsciously influences the personas we create, and in turn the systems we build. However, by embedding data intelligence in the way we design for people, we can ensure that no one is pigeonholed based on cultural assumptions. Statistical classification methods allow AI models to predict the probability that a data point belongs to a class based on its features, making those models useful tools for persona creation and customer segmentation.
To illustrate this, let’s look at an online grocery service. As an existing customer browses the e-commerce site, the associated trackable user behaviour could be used to train a model under unsupervised learning. By learning from data points across the end-to-end user journey, and linking these to the user’s personal characteristics, the AI could identify clusters and patterns: password forgetters, family basket builders, coupon lovers, etc. Furthermore, using a classification method such as the ‘K-nearest neighbours’ algorithm (k-NN), a newly-registered customer could even be categorised further by comparing their proximity with similar customers already in the database. These are our AI-influenced personas: living, breathing, up-to-date, behavioural-based groupings that represent a more accurate view of our audience.
Experience measurement
One of the greatest challenges that designers face in today’s business landscape is to measure and quantify the value of something as amorphous as an experience. But by working closely with engineers, we can train models to analyse the data points in an experience and generate a current-state experience score for a service as it occurs in real-time. Experiences are complex. In the example of a ride-hailing service such as Uber, deep neural networks would learn from a range of variables such as new daily account sign-ups, average wait time, newly opened support cases, driver star rating and even detected tone of voice in reviews. Intelligent scoring systems like these allow for the real-time measurement of experience, and to understand whether customers’ evolving needs and expectations are being met.
Furthermore, AI recommender systems can use collaborative filtering techniques to accurately predict which elements of an experience a customer is most likely to prefer based on comparable customers. These machine outputs could then be used to adapt the digital experience of each customer accordingly, to see if it yields a better experience score. Overall, having data-driven visibility over how a service is doing makes this an intelligent tool for the business to make decisions, for designers to map new service improvements, and for developers to prioritise feature backlogs. The great value trifecta! Without a doubt, the greatest advantage that AI poses to designers is the ability to learn faster, which is essential at the speed of today’s world.
How can service design help engineer better AI?
Engineering, like design, is all about problem-solving. As designers, we have a range of tried-and-tested techniques and creative methodologies that help us to think about problems in new ways.
Understanding the whole
When it comes to any new technology, we need to think about the unintended consequences, and, as Google’s CEO, Sundar Pichai puts it, “with AI, we need to think about it earlier”. AI is already being used for high-stakes decision-making, such as criminal sentencing. In complex use-cases such as these, service blueprints can help to visualise all the different people, processes, tools and systems involved from the moment a person is accused, through to their police arrest, sentencing journey, bail processes and possible rehabilitation back into society. By mapping the entire journey from end-to-end with engineers, we can identify pain points such as lack of clear legal communication, and potential risks in the data funnel such as societal racial biases. Aside from identifying pain points, mapping the whole user journey from end-to-end is also a creative way to uncover innovative use cases for AI. It is fair to say that AI has reached the peak of inflated expectations, but with a systems design lens, we can chart the next step: the slope of creativity.
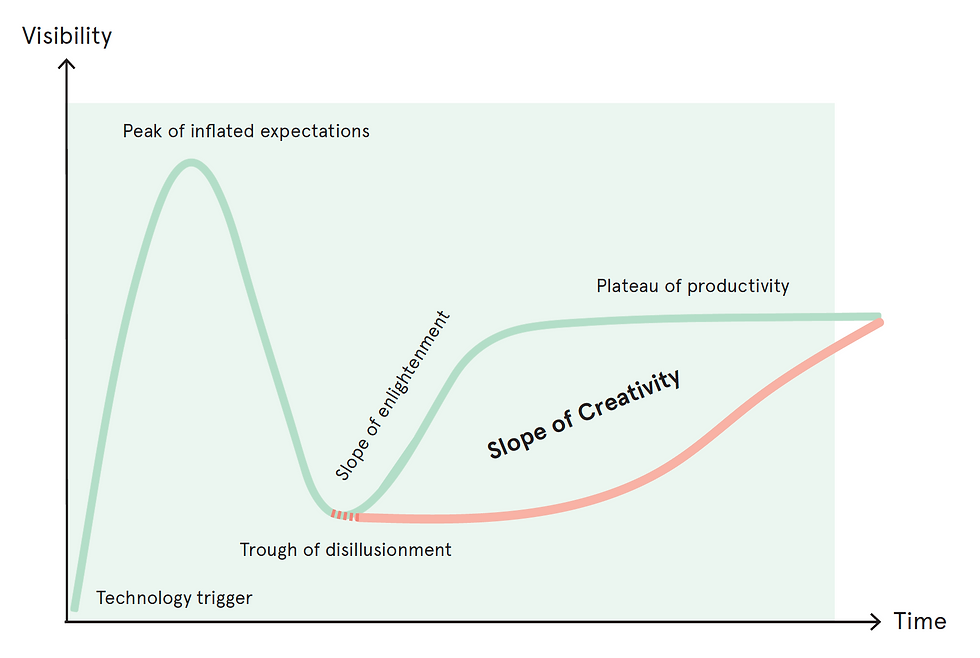
The slope of creativity is where we tell human-centred stories that make people’s lives better through technology. During this phase, mature organisations searching for meaningful value beyond industry hype are embracing design methodologies to unpack their customer journeys, organisational processes, supply chains and systems. They are mapping their businesses, identifying pain points and evaluating the roles that AI has to play in improving the human experiences they offer. This could mean using AI to provide personalised feedback to every single unsuccessful job applicant in a recruitment journey, or analysing shared medical records around the world to form interventions that can make entire geographies healthier. Towards the end of this phase, user adoption skyrockets as people start to perceive meaningful value in their day-today lives, and the technology becomes effortlessly interwoven with the reality of the human experience.
Explainable AI
AI is deeply based on statistical and numerical information. It can help us to make smarter decisions, but it needs design to make it explainable through empathy. Explainable AI as a service requires designers to ask, “But what does it mean for the people receiving these machine learning outputs?”. It calls us to work closely with engineers to inform the most user-centred data strategy possible. To put this into context, as we move towards AI-driven healthcare, it is probable that machine learning models will be used to determine which type of treatment a patient should receive. We will need well-designed guides that can empower doctors on how to interpret the outputs of the model (to understand where they came from and how these conclusions were reached), as well as how to use the outputs in combination with the living, breathing patient they see in front of them to make the right decision.
Empathetic design tools such as emotion-based experience mapping will be fundamental in helping teams to interpret and empathise with what a patient is feeling at particular moments of the journey, such as confusion, anxiety or financial concern. Furthermore, through visually storyboarding the journey of a patient at the hospital, we can understand how this information should be best communicated, and design for that outcome. As AI becomes embedded in our day-to-day lives, human empathy will be crucial in getting these experiences right.
Change co-created
Change management is a widely used term in digital transformation, but when it comes to AI we can’t manage change; we have to co-create it. Responsible development of AI will require strong private-to-public partnerships, increased collaboration across different sectors like healthcare and government, and working with academic institutions and non-profits. Law enforcement will have a key role to play in thoughtful regulation, and we will need to strike the right balance so there is still innovation and accountability. Collaboration across all these different stakeholders will be vital and is where design-led co-creation workshops will come in handy. These will help illustrate what intelligent journeys could look like, highlight cross-dependencies and gain alignment across different stakeholders.
For example, one of the most profound use cases of AI will be to solve the great labour shortage when it comes to the production and manufacturing of goods around the world. Working in cross-functional teams to co-create current-state journeys with factory workers, fulfilment managers and C-suite leaders will help us to understand how AI can actually improve operations on the ground. Design Thinking methodologies can help identify processes and levers for affected workers to be up-skilled and redeployed into more meaningful work, such as model observation, training or robotics maintenance roles in the supply chain. AI disruption will occur in every industry. The process of navigating that change ethically and inclusively will not happen on its own – it will need to be designed.
A symbiotic approach
Design needs AI to help augment our intelligence, reduce human bias and measure user experiences. AI needs design to help map the whole system, build from a point of empathy and co-create across stakeholders. The relationship between creativity and intelligence is symbiotic. With the exception of space exploration, AI is probably the greatest opportunity that we have to dramatically change the course of history and completely redefine the human experience. The question is, will we seize it?
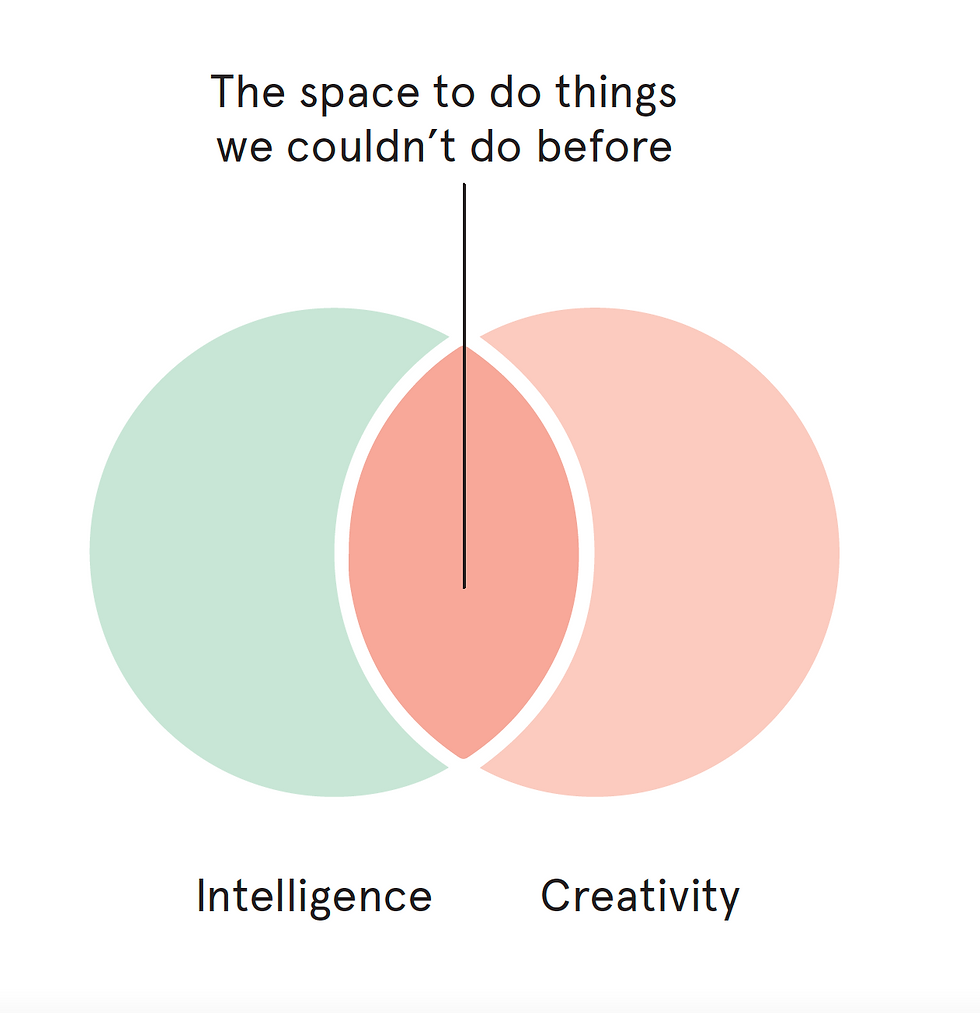
Getting started
In the US alone, 80 percent of service designers are employed by the technology sector. So, the good news? Most of us are already here. What’s left to do is to fight for a seat at the AI development tables of our own tech organisations. Be eager and willing to learn from ML experts. Ask questions. Read articles. Shadow model training. Look up things you don’t know. Be curious. Be bold, and proudly contribute to the data intelligence conversations happening on the ground where you are. The more closely intertwined design and engineering become, the more well-rounded our outputs and solutions will be. Bridging those siloes and blurring boundary lines is never easy. But without a doubt, the most important conversation to define the next century will be that of the one between creativity and intelligence. If it hasn’t yet begun, let us consider this the start.
Simbi Ladipo
Written and published 2023
SDN Touchpoint Vol. 13 No. 3 – Smart Service Design
Comments